Hossain, Mohammad Ishtiyaq and Amin, A. K. M. Nurul and Patwari, Muhammed Anayet Ullah
(2011)
Artificial neural network algorithm for predicting the surface roughness in end milling of Inconel 718 alloy.
In:
Advanced Machining Towards Improved Machinability of Difficult-to-Cut Materials.
IIUM university press, International Islamic University Malaysia, Kuala Lumpur, Malaysia, pp. 149-154.
ISBN 9789674181758
![[img]](http://irep.iium.edu.my/style/images/fileicons/application_pdf.png) 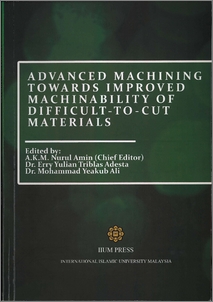 Preview |
|
PDF (Artificial neural network algorithm for predicting the surface roughness in end milling of Inconel 718 alloy)
- Published Version
Download (557kB)
| Preview
|
Abstract
Surface roughness is one of the important factors for evaluating workpiece quality during the
machining process because the quality of surface roughness affects the functional characteristics
of the workpiece such as compatibility, fatigue resistance and surface friction. The factors that
affect the surface roughness during the end milling process include tool geometry, feed rate,
depth of cut and cutting speed. Several researchers have studied the end milling process in the
recent years. The researchers also used response surface methodology (RSM) to explore the
effect of cutting parameters as cutting speed, feed rate and axial depth of cut. Alauddin et al. [1]
developed a mathematical model to predict the surface roughness of steel after end milling. The
prediction model was expressed via cutting speed, feed rate and depth of cut. Fuh and Hwang [2]
used RSM to construct a model that can predict the milling force in end milling operations. But
as the machining process is nonlinear and time-dependent, it is difficult for the traditional
identification methods to provide an accurate model. Compared to traditional computing
methods, the artificial neural networks (ANNs) are robust and global. ANNs have the
characteristics of universal approximation, parallel distributed processing, hardware
implementation, learning and adaptation, and multivariable systems [3]. ANNs have been
extensively applied in modeling many metal-cutting operations such as turning, milling, and
drilling [4-5]. However, this study was inspired by the very limited work on the application of
ANNs in modeling the relationship between cutting conditions and the surface roughness during
high-speed end milling of nickel-based, Inconel 718 alloy.
Actions (login required)
 |
View Item |